本文 github 地址:1-1 基本模型調(diào)用. ipynb放接,里面會(huì)記錄自己kaggle大賽中的內(nèi)容,歡迎start關(guān)注土匀。
cmd 地址:xgboost 庫(kù)使用入門
# 開啟多行顯示
from IPython.core.interactiveshell import InteractiveShell
InteractiveShell.ast_node_interactivity = "all"
# InteractiveShell.ast_node_interactivity = "last_expr"
# 顯示圖片
%matplotlib inline
%config InlineBackend.figure_format = 'retina'
數(shù)據(jù)探索
XGBoost中數(shù)據(jù)形式可以是libsvm的菊匿,libsvm作用是對(duì)稀疏特征進(jìn)行優(yōu)化城丧,看個(gè)例子:
1 101:1.2 102:0.03
0 1:2.1 10001:300 10002:400
0 2:1.2 1212:21 7777:2
每行表示一個(gè)樣本影锈,每行開頭0芹务,1表示標(biāo)簽,而后面的則是特征索引:數(shù)值鸭廷,其他未表示都是0.
我們以判斷蘑菇是否有毒為例子來做后續(xù)的訓(xùn)練枣抱。數(shù)據(jù)集來自:http://archive.ics.uci.edu/ml/machine-learning-databases/mushroom/ ,其中蘑菇有22個(gè)屬性辆床,將這些原始的特征加工后得到126維特征佳晶,并保存為libsvm格式,標(biāo)簽是表示蘑菇是否有毒讼载。其中其中 6513 個(gè)樣本做訓(xùn)練宵晚,1611 個(gè)樣本做測(cè)試。
import xgboost as xgb
from sklearn.metrics import accuracy_score
DMatrix is a internal data structure that used by XGBoost
which is optimized for both memory efficiency and training speed.
DMatrix 的數(shù)據(jù)來源可以是 string/numpy array/scipy.sparse/pd.DataFrame
维雇,如果是 string,則代表 libsvm 文件的路徑晒他,或者是 xgboost 可讀取的二進(jìn)制文件路徑吱型。
data_fold = "./data/"
dtrain = xgb.DMatrix(data_fold + "agaricus.txt.train")
dtest = xgb.DMatrix(data_fold + "agaricus.txt.test")
查看數(shù)據(jù)情況
(dtrain.num_col(),dtrain.num_row())
(dtest.num_col(),dtest.num_row())
(127, 6513)
(127, 1611)
模型訓(xùn)練
基本參數(shù)設(shè)定:
- max_depth: 樹的最大深度。缺省值為6陨仅,取值范圍為:[1,∞]
- eta:為了防止過擬合津滞,更新過程中用到的收縮步長(zhǎng)铝侵。eta通過縮減特征 的權(quán)重使提升計(jì)算過程更加保守。缺省值為0.3触徐,取值范圍為:[0,1]
- silent: 0表示打印出運(yùn)行時(shí)信息咪鲜,取1時(shí)表示以緘默方式運(yùn)行,不打印 運(yùn)行時(shí)信息撞鹉。缺省值為0
- objective: 定義學(xué)習(xí)任務(wù)及相應(yīng)的學(xué)習(xí)目標(biāo)疟丙,“binary:logistic” 表示 二分類的邏輯回歸問題,輸出為概率鸟雏。
param = {'max_depth':2, 'eta':1, 'silent':0, 'objective':'binary:logistic' }
%time
# 設(shè)置boosting迭代計(jì)算次數(shù)
num_round = 2
bst = xgb.train(param, dtrain, num_round)
CPU times: user 0 ns, sys: 0 ns, total: 0 ns
Wall time: 65.6 μs
此處模型輸出是一個(gè)概率值享郊,我們將其轉(zhuǎn)換為0-1值,然后再計(jì)算準(zhǔn)確率
train_preds = bst.predict(dtrain)
train_predictions = [round(value) for value in train_preds]
y_train = dtrain.get_label()
train_accuracy = accuracy_score(y_train, train_predictions)
print ("Train Accuary: %.2f%%" % (train_accuracy * 100.0))
Train Accuary: 97.77%
我們最后再測(cè)試集上看下模型的準(zhǔn)確率的
preds = bst.predict(dtest)
predictions = [round(value) for value in preds]
y_test = dtest.get_label()
test_accuracy = accuracy_score(y_test, predictions)
print("Test Accuracy: %.2f%%" % (test_accuracy * 100.0))
Test Accuracy: 97.83%
from matplotlib import pyplot
import graphviz
xgb.to_graphviz(bst, num_trees=0 )
pyplot.show()
scikit-learn 接口格式
from xgboost import XGBClassifier
from sklearn.datasets import load_svmlight_file
my_workpath = './data/'
X_train,y_train = load_svmlight_file(my_workpath + 'agaricus.txt.train')
X_test,y_test = load_svmlight_file(my_workpath + 'agaricus.txt.test')
# 設(shè)置boosting迭代計(jì)算次數(shù)
num_round = 2
#bst = XGBClassifier(**params)
#bst = XGBClassifier()
bst =XGBClassifier(max_depth=2, learning_rate=1, n_estimators=num_round,
silent=True, objective='binary:logistic')
bst.fit(X_train, y_train)
XGBClassifier(base_score=0.5, booster='gbtree', colsample_bylevel=1,
colsample_bytree=1, gamma=0, learning_rate=1, max_delta_step=0,
max_depth=2, min_child_weight=1, missing=None, n_estimators=2,
n_jobs=1, nthread=None, objective='binary:logistic', random_state=0,
reg_alpha=0, reg_lambda=1, scale_pos_weight=1, seed=None,
silent=True, subsample=1)
# 訓(xùn)練集上準(zhǔn)確率
train_preds = bst.predict(X_train)
train_predictions = [round(value) for value in train_preds]
train_accuracy = accuracy_score(y_train, train_predictions)
print ("Train Accuary: %.2f%%" % (train_accuracy * 100.0))
Train Accuary: 97.77%
# 測(cè)試集上準(zhǔn)確率
# make prediction
preds = bst.predict(X_test)
predictions = [round(value) for value in preds]
test_accuracy = accuracy_score(y_test, predictions)
print("Test Accuracy: %.2f%%" % (test_accuracy * 100.0))
Test Accuracy: 97.83%
scikit-lean 中 cv 使用
做cross_validation主要用到下面 StratifiedKFold 函數(shù)
# 設(shè)置boosting迭代計(jì)算次數(shù)
num_round = 2
bst =XGBClassifier(max_depth=2, learning_rate=0.1,n_estimators=num_round,
silent=True, objective='binary:logistic')
from sklearn.model_selection import StratifiedKFold
from sklearn.model_selection import cross_val_score
kfold = StratifiedKFold(n_splits=10, random_state=7)
results = cross_val_score(bst, X_train, y_train, cv=kfold)
print(results)
print("CV Accuracy: %.2f%% (%.2f%%)" % (results.mean()*100, results.std()*100))
[ 0.69478528 0.85276074 0.95398773 0.97235023 0.96006144 0.98771121
1. 1. 0.96927803 0.97695853]
CV Accuracy: 93.68% (9.00%)
GridSearchcv 搜索最優(yōu)解
from sklearn.model_selection import GridSearchCV
bst =XGBClassifier(max_depth=2, learning_rate=0.1, silent=True, objective='binary:logistic')
%time
param_grid = {
'n_estimators': range(1, 51, 1)
}
clf = GridSearchCV(bst, param_grid, "accuracy",cv=5)
clf.fit(X_train, y_train)
CPU times: user 0 ns, sys: 0 ns, total: 0 ns
Wall time: 24.3 μs
clf.best_params_, clf.best_score_
({'n_estimators': 30}, 0.98418547520343924)
## 在測(cè)試集合上測(cè)試
#make prediction
preds = clf.predict(X_test)
predictions = [round(value) for value in preds]
test_accuracy = accuracy_score(y_test, predictions)
print("Test Accuracy of gridsearchcv: %.2f%%" % (test_accuracy * 100.0))
Test Accuracy of gridsearchcv: 97.27%
early-stop
我們?cè)O(shè)置驗(yàn)證valid集孝鹊,當(dāng)我們迭代過程中發(fā)現(xiàn)在驗(yàn)證集上錯(cuò)誤率增加炊琉,則提前停止迭代。
from sklearn.model_selection import train_test_split
seed = 7
test_size = 0.33
X_train_part, X_validate, y_train_part, y_validate= train_test_split(X_train, y_train, test_size=test_size,
random_state=seed)
X_train_part.shape
X_validate.shape
(4363, 126)
(2150, 126)
# 設(shè)置boosting迭代計(jì)算次數(shù)
num_round = 100
bst =XGBClassifier(max_depth=2, learning_rate=0.1, n_estimators=num_round, silent=True, objective='binary:logistic')
eval_set =[(X_validate, y_validate)]
bst.fit(X_train_part, y_train_part, early_stopping_rounds=10, eval_metric="error",
eval_set=eval_set, verbose=True)
[0] validation_0-error:0.048372
Will train until validation_0-error hasn't improved in 10 rounds.
[1] validation_0-error:0.042326
[2] validation_0-error:0.048372
[3] validation_0-error:0.042326
[4] validation_0-error:0.042326
[5] validation_0-error:0.042326
[6] validation_0-error:0.023256
[7] validation_0-error:0.042326
[8] validation_0-error:0.042326
[9] validation_0-error:0.023256
[10] validation_0-error:0.006512
[11] validation_0-error:0.017674
[12] validation_0-error:0.017674
[13] validation_0-error:0.017674
[14] validation_0-error:0.017674
[15] validation_0-error:0.017674
[16] validation_0-error:0.017674
[17] validation_0-error:0.017674
[18] validation_0-error:0.024651
[19] validation_0-error:0.020465
[20] validation_0-error:0.020465
Stopping. Best iteration:
[10] validation_0-error:0.006512
我們可以將上面的錯(cuò)誤率進(jìn)行可視化又活,方便我們更直觀的觀察
results = bst.evals_result()
#print(results)
epochs = len(results['validation_0']['error'])
x_axis = range(0, epochs)
# plot log loss
fig, ax = pyplot.subplots()
ax.plot(x_axis, results['validation_0']['error'], label='Test')
ax.legend()
pyplot.ylabel('Error')
pyplot.xlabel('Round')
pyplot.title('XGBoost Early Stop')
pyplot.show()
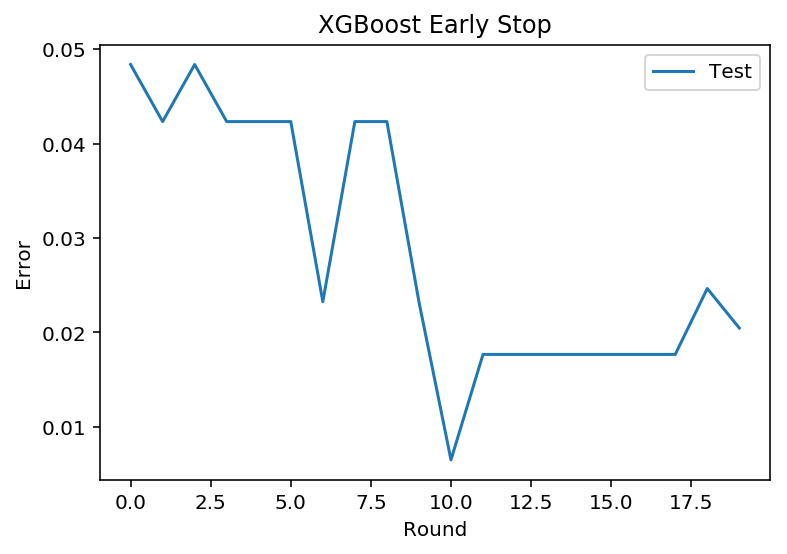
# 測(cè)試集上準(zhǔn)確率
# make prediction
preds = bst.predict(X_test)
predictions = [round(value) for value in preds]
test_accuracy = accuracy_score(y_test, predictions)
print("Test Accuracy: %.2f%%" % (test_accuracy * 100.0))
Test Accuracy: 97.27%
學(xué)習(xí)曲線
# 設(shè)置boosting迭代計(jì)算次數(shù)
num_round = 100
# 沒有 eraly_stop
bst =XGBClassifier(max_depth=2, learning_rate=0.1, n_estimators=num_round, silent=True, objective='binary:logistic')
eval_set = [(X_train_part, y_train_part), (X_validate, y_validate)]
bst.fit(X_train_part, y_train_part, eval_metric=["error", "logloss"], eval_set=eval_set, verbose=True)
# retrieve performance metrics
results = bst.evals_result()
#print(results)
epochs = len(results['validation_0']['error'])
x_axis = range(0, epochs)
# plot log loss
fig, ax = pyplot.subplots()
ax.plot(x_axis, results['validation_0']['logloss'], label='Train')
ax.plot(x_axis, results['validation_1']['logloss'], label='Test')
ax.legend()
pyplot.ylabel('Log Loss')
pyplot.title('XGBoost Log Loss')
pyplot.show()
# plot classification error
fig, ax = pyplot.subplots()
ax.plot(x_axis, results['validation_0']['error'], label='Train')
ax.plot(x_axis, results['validation_1']['error'], label='Test')
ax.legend()
pyplot.ylabel('Classification Error')
pyplot.title('XGBoost Classification Error')
pyplot.show()
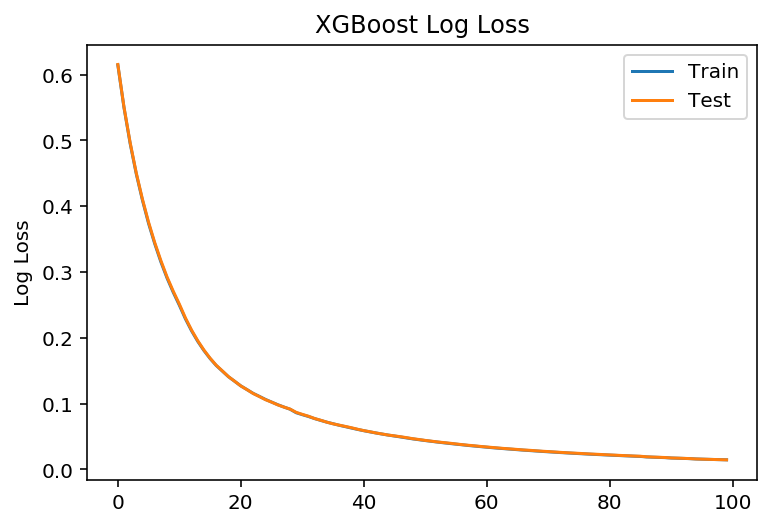
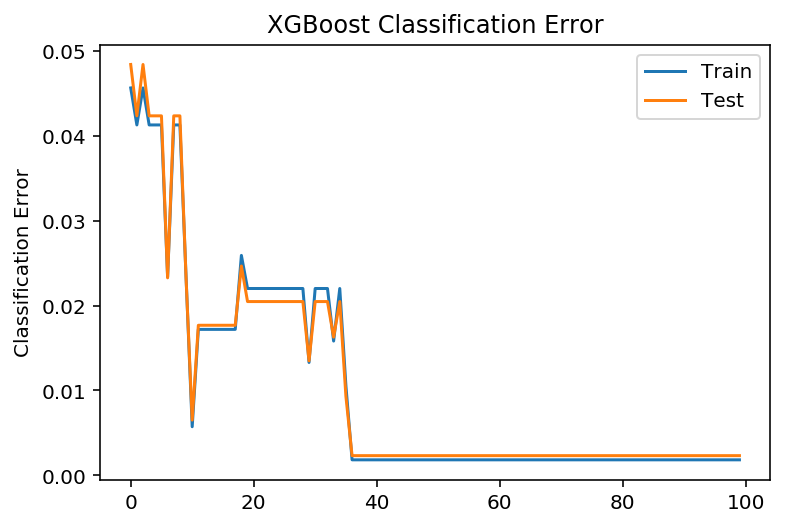
# make prediction
preds = bst.predict(X_test)
predictions = [round(value) for value in preds]
test_accuracy = accuracy_score(y_test, predictions)
print("Test Accuracy: %.2f%%" % (test_accuracy * 100.0))
Test Accuracy: 99.81%