Recurrent Neural Networks Tutorial, Part 1 – Introduction to RNNs
Recurrent Neural Networks (RNNs) are popular models that have shown great promise in many NLP tasks. But despite their recent popularity I’ve only found a limited number of resources that throughly explain how RNNs work, and how to implement them. That’s what this tutorial is about. It’s a multi-part series in which I’m planning to cover the following:
RNN在NLP領(lǐng)域應(yīng)用廣泛掉缺,但是很少有文章能夠透徹的講解其原理攀圈,更別提用精煉的代碼實現(xiàn)出來峦甩。這篇教程就是干這個的现喳,因為內(nèi)容比較多嗦篱,分成四個部分。
- Introduction to RNNs (this post)
- Implementing a RNN using Python and Theano
- Understanding the Backpropagation Through Time (BPTT) algorithm and the vanishing gradient problem
- Implementing a GRU/LSTM RNN
As part of the tutorial we will implement a recurrent neural network based language model. 我們要用RNN實現(xiàn)一個語言模型.
The applications of language models are two-fold: First, it allows us to score arbitrary sentences based on how likely they are to occur in the real world. This gives us a measure of grammatical and semantic correctness. Such models are typically used as part of Machine Translation systems. 這種模型的典型用途之一是機器翻譯诫欠。Secondly, a language model allows us to generate new text (I think that’s the much cooler application). Training a language model on Shakespeare allows us to generate Shakespeare-like text. 另一種用途是生成文章荒叼,比如機器看了莎士比亞全集典鸡,然后模仿寫出一篇模仿莎士比亞的文章萝玷。This fun post by Andrej Karpathy demonstrates what character-level language models based on RNNs are capable of.
I'm assuming that you are somewhat familiar with basic Neural Networks. If you’re not, you may want to head over to Implementing A Neural Network From Scratch, which guides you through the ideas and implementation behind non-recurrent networks.
這篇文章要求讀者有基本的NN知識昆婿。
What are RNNs?
問題來了仓蛆,什么是RNN?
The idea behind RNNs is to make use of sequential information. RNN的思想是利用信息的連續(xù)性法精。In a traditional neural network we assume that all inputs (and outputs) are independent of each other. But for many tasks that’s a very bad idea. If you want to predict the next word in a sentence you better know which words came before it. 原始的NN模型利用的是信息的獨立性搂蜓,但是在很多方面受限,比如要預測句子缺的一個單詞相味,最好要先知道句子的結(jié)構(gòu)或者前一個單詞殉挽。RNNs are called recurrent because they perform the same task for every element of a sequence, with the output being depended on the previous computations. Another way to think about RNNs is that they have a “memory” which captures information about what has been calculated so far. In theory RNNs can make use of information in arbitrarily long sequences, but in practice they are limited to looking back only a few steps (more on this later). RNN實現(xiàn)的是一種回頭看的機制斯碌,也就是具有記憶功能,我要預測下一個單詞投慈,我要了解這個句子冠骄。Here is what a typical RNN looks like:
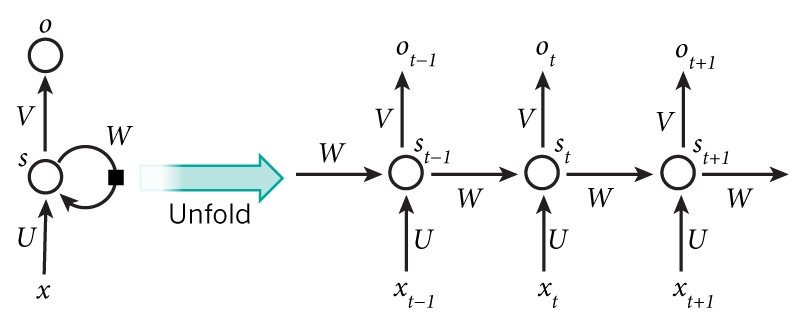
A recurrent neural network and the unfolding in time of the computation involved in its forward computation. Source: Nature
圖中表示了一個RNN 神經(jīng)元的經(jīng)典表述凛辣。
The above diagram shows a RNN being unrolled (or unfolded) into a full network. By unrolling we simply mean that we write out the network for the complete sequence. 上圖展示了展開的RNN扁誓,所謂展開,意思是說整個網(wǎng)絡(luò)的序列性被顯示出來捷泞。比如說前普,如果我們的句子里邊有五個單詞,這個序列會被展開成一個五層的網(wǎng)絡(luò) 骡湖。For example, if the sequence we care about is a sentence of 5 words, the network would be unrolled into a 5-layer neural network, one layer for each word. The formulas that govern the computation happening in a RNN are as follows:
xt is the input at time step t. For example, could be a one-hot vector corresponding to the second word of a sentence. Xt 是t關(guān)于時間的輸入响蕴,比如可以是一個詞向量。
St is the hidden state at time step t. It's the “memory” of the network. St is calculated based on the previous hidden state and the input at the current step: . St 是在t的隱藏狀態(tài)辖试,計算它要以來當前的輸入和上一個隱藏狀態(tài)的值罐孝。The function f usually is a nonlinearity such as tanh or ReLU. S-1, which is required to calculate the first hidden state, is typically initialized to all zeroes.跟普通NN一樣莲兢,激活函數(shù)是非線性的续膳,S-1隱藏狀態(tài)被初始化為0。
Ot is the output at step t. For example, if we wanted to predict the next word in a sentence it would be a vector of probabilities across our vocabulary. .Ot是輸出谒兄,比如要預測的單詞的輸出就是單詞表各個單詞的概率
There are a few things to note here:
You can think of the hidden state 'St' as the memory of the network. St captures information about what happened in all the previous time steps. St 可以理解為網(wǎng)絡(luò)的存儲器承疲,它保存了網(wǎng)絡(luò)之前的所以信息瘦穆。The output Ot at step t is calculated solely based on the memory at time t. As briefly mentioned above, it’s a bit more complicated in practice because typically St can’t capture information from too many time steps ago.Ot 只依賴t時刻的St, 但是實踐中扛或,St想反應(yīng)所有之前的狀態(tài)信息是很困難的碘饼,因為需要向回看的步驟太多了艾恼。
Unlike a traditional deep neural network, which uses different parameters at each layer, a RNN shares the same parameters ( above) across all steps. This reflects the fact that we are performing the same task at each step, just with different inputs. This greatly reduces the total number of parameters we need to learn.之前的NN需要每層都都不同的算法,參數(shù)也各異舆声,但是RNN的每一層只需要相同的參數(shù)。這也就意味著需要的參數(shù)很少碱屁,每一步的輸入不同蛾找,但是作用相同打毛。
The above diagram has outputs at each time step, but depending on the task this may not be necessary. For example, when predicting the sentiment of a sentence we may only care about the final output, not the sentiment after each word. 并不是每一步都需要輸出的,模型可以是一對多碰声,多對多奥邮。同樣的也不是每一步都需要輸入罗珍。 Similarly, we may not need inputs at each time step. The main feature of an RNN is its hidden state, which captures some information about a sequence.現(xiàn)在就可以看出RNN的本質(zhì)特性就在于隱藏狀態(tài)可以反映序列的信息。比如蘸朋,時間
What can RNNs do?
RNNs have shown great success in many NLP tasks. At this point I should mention that the most commonly used type of RNNs are LSTMs, which are much better at capturing long-term dependencies than vanilla RNNs are. But don’t worry, LSTMs are essentially the same thing as the RNN we will develop in this tutorial, they just have a different way of computing the hidden state. We’ll cover LSTMs in more detail in a later post. Here are some example applications of RNNs in NLP (by non means an exhaustive list).下面介紹NLP領(lǐng)域的應(yīng)用藕坯。
Language Modeling and Generating Text
Given a sequence of words we want to predict the probability of each word given the previous words. Language Models allow us to measure how likely a sentence is, which is an important input for Machine Translation (since high-probability sentences are typically correct). A side-effect of being able to predict the next word is that we get a generative model, which allows us to generate new text by sampling from the output probabilities. And depending on what our training data is we can generate all kinds of stuff. In Language Modeling our input is typically a sequence of words (encoded as one-hot vectors for example), and our output is the sequence of predicted words. When training the network we set since we want the output at step to be the actual next word.
Research papers about Language Modeling and Generating Text:
Recurrent neural network based language model
Extensions of Recurrent neural network based language model
Generating Text with Recurrent Neural Networks
Machine Translation
Machine Translation is similar to language modeling in that our input is a sequence of words in our source language (e.g. German). We want to output a sequence of words in our target language (e.g. English). A key difference is that our output only starts after we have seen the complete input, because the first word of our translated sentences may require information captured from the complete input sequence.
RNN for Machine Translation. Image Source: http://cs224d.stanford.edu/lectures/CS224d-Lecture8.pdf
Research papers about Machine Translation:
A Recursive Recurrent Neural Network for Statistical Machine Translation
Sequence to Sequence Learning with Neural Networks
Joint Language and Translation Modeling with Recurrent Neural Networks
Speech Recognition
Given an input sequence of acoustic signals from a sound wave, we can predict a sequence of phonetic segments together with their probabilities.
Research papers about Speech Recognition:
Towards End-to-End Speech Recognition with Recurrent Neural Networks
Generating Image Descriptions
Together with convolutional Neural Networks, RNNs have been used as part of a model to generate descriptions for unlabeled images. It’s quite amazing how well this seems to work. The combined model even aligns the generated words with features found in the images.
Deep Visual-Semantic Alignments for Generating Image Descriptions. Source: http://cs.stanford.edu/people/karpathy/deepimagesent/
Training RNNs
Training a RNN is similar to training a traditional Neural Network. We also use the backpropagation algorithm, but with a little twist. 都用BP訓練Because the parameters are shared by all time steps in the network, the gradient at each output depends not only on the calculations of the current time step, but also the previous time steps.但是RNN的梯度不只依賴輸出辐马,還依賴上一個狀態(tài)局义。 For example, in order to calculate the gradient at t = 4 we would need to backpropagate 3 steps and sum up the gradients. 比如t4時刻的梯度等于前三步bp的累加。 This is called Backpropagation Through Time (BPTT). If this doesn’t make a whole lot of sense yet, don’t worry, we’ll have a whole post on the gory details. For now, just be aware of the fact that vanilla RNNs trained with BPTT have difficulties learning long-term dependencies (e.g. dependencies between steps that are far apart) due to what is called the vanishing/exploding gradient problem. 長期訓練會有梯度消失檩帐,梯度爆炸等問題There exists some machinery to deal with these problems, and certain types of RNNs (like LSTMs) were specifically designed to get around them.用不同的網(wǎng)絡(luò)類型解決這個問題湃密,比較出名的是LTSM
RNN Extensions
Over the years researchers have developed more sophisticated types of RNNs to deal with some of the shortcomings of the vanilla RNN model. We will cover them in more detail in a later post, but I want this section to serve as a brief overview so that you are familiar with the taxonomy of models.
Bidirectional RNNs are based on the idea that the output at time may not only depend on the previous elements in the sequence, but also future elements. 雙向RNN解決未來依賴問題 For example, to predict a missing word in a sequence you want to look at both the left and the right context. 比如填詞游戲泛源,不僅要看左邊還要看右邊 Bidirectional RNNs are quite simple. They are just two RNNs stacked on top of each other. The output is then computed based on the hidden state of both RNNs.
Deep (Bidirectional) RNNs are similar to Bidirectional RNNs, only that we now have multiple layers per time step. In practice this gives us a higher learning capacity (but we also need a lot of training data).深度雙向RNN每一步都有多個層俩由,因此容量很大
LSTM networks are quite popular these days and we briefly talked about them above. LSTMs don’t have a fundamentally different architecture from RNNs, but they use a different function to compute the hidden state.LSTM是目前最流行的一種了,但是唯一的差別是計算隱藏層狀態(tài)的函數(shù)不一樣兜畸。 The memory in LSTMs are called cells and you can think of them as black boxes that take as input the previous state and current inputLSTM的memory叫做cell碘梢,cell負責處理前面的狀態(tài)和輸入 . Internally these cells decide what to keep in (and what to erase from) memorycell內(nèi)部自己決定是從往內(nèi)存記錄還說擦除信息. They then combine the previous state, the current memory, and the inputcell會合并前面的狀態(tài)煞躬,當前的內(nèi)存和輸入。. It turns out that these types of units are very efficient at capturing long-term dependencies. 事實證明LSTM在記錄長期依賴信息時很有效 LSTMs can be quite confusing in the beginning but if you’re interested in learning more this post has an excellent explanation.剛開始理解LSTM比較困難在扰,但是會越來越有趣芒珠。
Conclusion
So far so good. I hope you’ve gotten a basic understanding of what RNNs are and what they can do. In the next post we’ll implement a first version of our language model RNN using Python and Theano. Please leave questions in the comments!
這篇文章主要是背景介紹搅裙,讀起來沒啥意思。