本文是seq2seq模型的第一篇催式,本文根據(jù)論文Sutskever, Vinyals and Le (2014)而來
github地址:https://github.com/zhuanxuhit/nd101/blob/master/1.Intro_to_Deep_Learning/11.How_to_Make_a_Language_Translator/1-seq2seq.ipynb
參考的文章:https://github.com/ematvey/tensorflow-seq2seq-tutorials/blob/master/1-seq2seq.ipynb
import helper
看圖:
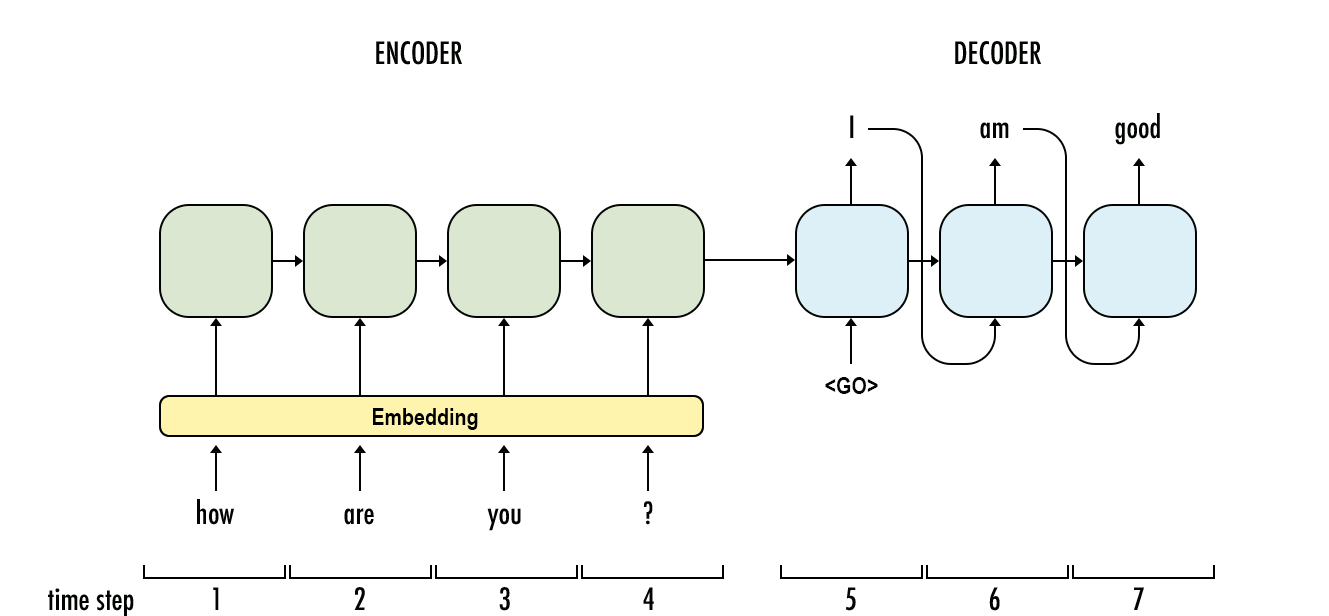
在實(shí)際的word2word中趣避,我們會(huì)對(duì)單詞進(jìn)行embedding操作,此處為了簡(jiǎn)單起見,我們直接就以數(shù)字代表輸入了
x = [[5, 7, 8], [6, 3], [3], [1]]
xt, xlen = helper.batch(x)
xt # [max_time_len, batch_size]
array([[5, 6, 3, 1],
[7, 3, 0, 0],
[8, 0, 0, 0]], dtype=int32)
xlen
[3, 2, 1, 1]
在處理中逻住,我們會(huì)做一些特殊的處理
- < PAD>: 在訓(xùn)練過程中创葡,batch中每個(gè)句子長(zhǎng)度會(huì)不同了,此時(shí)我們對(duì)于短的就直接用 < PAD> 來填充的
- < EOS>: EOS代表的句子的結(jié)尾
- < UNK>: 對(duì)于一些不常見的詞匯唠叛,直接用UNK替換掉(例如人名)
- < GO>: decode的第一個(gè)輸入只嚣,告訴decode預(yù)測(cè)開始
定義模型
在定義模型的時(shí)候,我們需要確定的是 vocab_size 艺沼, input_embedding_size 和 encoder_hidden_units 和 decoder_hidden_units 册舞,一旦修改得重新定義模型
import tensorflow as tf
import numpy as np
PAD = 0
EOS = 1
# UNK = 2
# GO = 3
vocab_size = 10
input_embedding_size = 20
encoder_hidden_units = 20
decoder_hidden_units = encoder_hidden_units
對(duì)于一個(gè)復(fù)雜的模型,我們想要去了解他障般,最好的方式就是看輸入和輸出调鲸,seq2seq的模型其輸入和輸出是:
- encoder_inputs int32 tensor is shaped [encoder_max_time, batch_size]
- decoder_targets int32 tensor is shaped [decoder_max_time, batch_size]
encoder_inputs = tf.placeholder(shape=(None, None), dtype=tf.int32, name='encoder_inputs')
decoder_targets = tf.placeholder(shape=(None, None), dtype=tf.int32, name='decoder_targets')
我們還需要定義的一個(gè)輸入是decoder的輸入
- decoder_inputs int32 tensor is shaped [decoder_max_time, batch_size]
decoder_inputs = tf.placeholder(shape=(None, None), dtype=tf.int32, name='decoder_inputs')
在模型訓(xùn)練的時(shí)候,對(duì)于decoder的ouputs我們不會(huì)使用挽荡,而是直接使用decoder_targets作為decoder的輸入藐石,但是在做predictions的時(shí)候,我們卻會(huì)使用decoder的輸出作為下一個(gè)lstm的輸入定拟,這可能會(huì)引入 distribution shift from training to prediction.
Embeddings
我們系統(tǒng)的輸入encoder_inputs和decoder_inputs都是 [decoder_max_time, batch_size]的形狀于微,但是我們 encoder 和 decoder 的輸入形狀都是要 [max_time, batch_size, input_embedding_size], 因此我們需要對(duì)我們的是輸入做一個(gè)word embedded
embeddings = tf.Variable(tf.truncated_normal([vocab_size, input_embedding_size], mean=0.0, stddev=0.1), dtype=tf.float32)
encoder_inputs_embedded = tf.nn.embedding_lookup(embeddings, encoder_inputs)
decoder_inputs_embedded = tf.nn.embedding_lookup(embeddings, decoder_inputs)
print(encoder_inputs_embedded)
Tensor("embedding_lookup:0", shape=(?, ?, 20), dtype=float32)
encoder
encoder_cell = tf.contrib.rnn.BasicLSTMCell(encoder_hidden_units)
lstm_layers = 4
cell = tf.contrib.rnn.MultiRNNCell([encoder_cell] * lstm_layers)
# If `time_major == True`, this must be a `Tensor` of shape:
# `[max_time, batch_size, ...]`, or a nested tuple of such
# elements.
encoder_outputs, encoder_final_state = tf.nn.dynamic_rnn(cell,encoder_inputs_embedded,dtype=tf.float32,time_major=True)
del encoder_outputs
此處我們直接刪除了 encoder_outputs, 因?yàn)樵谶@個(gè)場(chǎng)景中我們是不關(guān)注的株依,我們需要的是最后的 encoder_final_state驱证,這又被稱為 "thought vector",如果沒有引入attention機(jī)制勺三,encoder_final_state 就是decoder的唯一輸入雷滚,用他來作為decoder的init_state來解出decoder_targets。
We hope that backpropagation through time (BPTT) algorithm will tune the model to pass enough information throught the thought vector for correct sequence output decoding.
print(encoder_final_state)
(LSTMStateTuple(c=<tf.Tensor 'rnn/while/Exit_2:0' shape=(?, 20) dtype=float32>, h=<tf.Tensor 'rnn/while/Exit_3:0' shape=(?, 20) dtype=float32>), LSTMStateTuple(c=<tf.Tensor 'rnn/while/Exit_4:0' shape=(?, 20) dtype=float32>, h=<tf.Tensor 'rnn/while/Exit_5:0' shape=(?, 20) dtype=float32>), LSTMStateTuple(c=<tf.Tensor 'rnn/while/Exit_6:0' shape=(?, 20) dtype=float32>, h=<tf.Tensor 'rnn/while/Exit_7:0' shape=(?, 20) dtype=float32>), LSTMStateTuple(c=<tf.Tensor 'rnn/while/Exit_8:0' shape=(?, 20) dtype=float32>, h=<tf.Tensor 'rnn/while/Exit_9:0' shape=(?, 20) dtype=float32>))
decoder
decoder_cell = tf.contrib.rnn.BasicLSTMCell(decoder_hidden_units)
decoder = tf.contrib.rnn.MultiRNNCell([decoder_cell] * lstm_layers)
decoder_outputs, decoder_final_state = tf.nn.dynamic_rnn(
decoder, decoder_inputs_embedded,
initial_state=encoder_final_state,
dtype=tf.float32, time_major=True, scope="plain_decoder",
)
此處我們不關(guān)心decoder_inputs吗坚,而是關(guān)心decoder_outputs祈远,對(duì)于decoder_outputs我們加一個(gè)fc,active_function=softmax商源,得到預(yù)測(cè)的單詞
decoder_logits = tf.contrib.layers.fully_connected(decoder_outputs,vocab_size,activation_fn=None,
weights_initializer = tf.truncated_normal_initializer(stddev=0.1),
biases_initializer=tf.zeros_initializer())
# decoder_prediction = tf.argmax(decoder_logits,)
print(decoder_logits)
Tensor("fully_connected/Reshape_1:0", shape=(?, ?, 10), dtype=float32)
decoder_prediction = tf.argmax(decoder_logits,2) # 在這一步我突然意識(shí)到了axis的含義车份。。牡彻。表明的竟然是在哪個(gè)維度上求 argmax扫沼。
print(decoder_prediction)
Tensor("ArgMax:0", shape=(?, ?), dtype=int64)
對(duì)于RNN的輸出,其shape是:[max_time, batch_size, hidden_units]庄吼,通過一個(gè)FC缎除,將其映射為:[max_time, batch_size, vocab_size]
# learn_rate = tf.placeholder(tf.float32)
stepwise_cross_entropy = tf.nn.softmax_cross_entropy_with_logits(
labels=tf.one_hot(decoder_targets, depth=vocab_size, dtype=tf.float32),
logits=decoder_logits,
)
loss = tf.reduce_mean(stepwise_cross_entropy)
train_op = tf.train.AdamOptimizer().minimize(loss)
試運(yùn)行
deep learning is a game of shapes
當(dāng)我們build graph的時(shí)候,如果shape錯(cuò)誤就馬上會(huì)提示总寻,但是一些其他的shape檢查器罐,只有我們運(yùn)行的時(shí)候才會(huì)發(fā)現(xiàn)錯(cuò)誤
with tf.Session() as sess:
sess.run(tf.global_variables_initializer())
batch_ = [[6], [3, 4], [9, 8, 7]]
batch_, batch_length_ = helper.batch(batch_)
print('batch_encoded:\n' + str(batch_))
din_, dlen_ = helper.batch(np.ones(shape=(3, 1), dtype=np.int32),
max_sequence_length=4)
print('decoder inputs:\n' + str(din_))
pred_ = sess.run(decoder_prediction,
feed_dict={
encoder_inputs: batch_,
decoder_inputs: din_,
# learn_rate:0.1,
})
print('decoder predictions:\n' + str(pred_))
print("build graph ok!")
batch_encoded:
[[6 3 9]
[0 4 8]
[0 0 7]]
decoder inputs:
[[1 1 1]
[0 0 0]
[0 0 0]
[0 0 0]]
decoder predictions:
[[9 6 6]
[9 6 2]
[9 6 2]
[9 9 4]]
build graph ok!
模擬訓(xùn)練
我們?yōu)榱撕?jiǎn)單起見,產(chǎn)生了隨機(jī)的輸入序列渐行,然后decoder原模原樣的輸出
batch_size = 100
batches = helper.random_sequences(length_from=3, length_to=8,
vocab_lower=2, vocab_upper=10,
batch_size=batch_size)
print('head of the batch:')
for seq in next(batches)[:10]:
print(seq)
head of the batch:
[7, 2, 9, 2, 2, 4, 4]
[6, 9, 8, 5, 2, 3]
[9, 3, 2, 4, 7]
[2, 5, 3, 3, 6, 8, 9]
[2, 4, 8, 5, 5, 3]
[2, 6, 3]
[3, 5, 2, 2]
[9, 5, 3]
[8, 5, 4, 2]
[4, 9, 5, 2, 4, 9]
def next_feed():
batch = next(batches)
encoder_inputs_, _ = helper.batch(batch)
decoder_targets_, _ = helper.batch(
[(sequence) + [EOS] for sequence in batch]
)
decoder_inputs_, _ = helper.batch(
[[EOS] + (sequence) for sequence in batch]
)
return {
encoder_inputs: encoder_inputs_,
decoder_inputs: decoder_inputs_,
decoder_targets: decoder_targets_,
}
當(dāng)encoder_inputs 是[5, 6, 7]是decoder_targets是 [5, 6, 7, 1],1代表的是EOF轰坊,decoder_inputs則是 [1, 5, 6, 7]
loss_track = []
max_batches = 3001
batches_in_epoch = 1000
with tf.Session() as sess:
sess.run(tf.global_variables_initializer())
try:
for batch in range(max_batches):
fd = next_feed()
_, l = sess.run([train_op, loss], fd)
loss_track.append(l)
if batch == 0 or batch % batches_in_epoch == 0:
print('batch {}'.format(batch))
print(' minibatch loss: {}'.format(sess.run(loss, fd)))
predict_ = sess.run(decoder_prediction, fd)
for i, (inp, pred) in enumerate(zip(fd[encoder_inputs].T, predict_.T)):
print(' sample {}:'.format(i + 1))
print(' input > {}'.format(inp))
print(' predicted > {}'.format(pred))
if i >= 2:
break
print()
except KeyboardInterrupt:
print('training interrupted')
batch 0
minibatch loss: 2.301229476928711
sample 1:
input > [4 8 3 3 4 8 0 0]
predicted > [0 0 0 0 0 0 0 0 0]
sample 2:
input > [4 8 7 8 4 3 0 0]
predicted > [0 0 0 0 0 0 0 0 0]
sample 3:
input > [6 4 3 0 0 0 0 0]
predicted > [6 0 0 0 0 0 0 0 0]
batch 1000
minibatch loss: 0.958212673664093
sample 1:
input > [7 2 6 8 0 0 0 0]
predicted > [7 7 3 3 1 0 0 0 0]
sample 2:
input > [2 6 8 6 3 8 0 0]
predicted > [3 3 6 6 6 6 1 0 0]
sample 3:
input > [5 2 4 4 0 0 0 0]
predicted > [5 4 4 4 1 0 0 0 0]
batch 2000
minibatch loss: 0.3982703983783722
sample 1:
input > [8 7 8 0 0 0 0 0]
predicted > [8 7 8 1 0 0 0 0 0]
sample 2:
input > [3 7 9 5 3 0 0 0]
predicted > [3 7 8 5 9 1 0 0 0]
sample 3:
input > [2 8 9 2 0 0 0 0]
predicted > [2 3 9 2 1 0 0 0 0]
batch 3000
minibatch loss: 0.27779871225357056
sample 1:
input > [3 4 5 4 3 8 4 0]
predicted > [3 4 4 5 3 2 4 1 0]
sample 2:
input > [5 4 6 3 8 0 0 0]
predicted > [5 4 6 3 2 1 0 0 0]
sample 3:
input > [4 7 6 0 0 0 0 0]
predicted > [4 7 6 1 0 0 0 0 0]
%matplotlib inline
import matplotlib.pyplot as plt
plt.plot(loss_track)
print('loss {:.4f} after {} examples (batch_size={})'.format(loss_track[-1], len(loss_track)*batch_size, batch_size))
loss 0.2582 after 300100 examples (batch_size=100)