示例怎么能夠產(chǎn)生一幅藝術(shù)
github閱讀效果更佳
假設(shè)我們有一幅大師的畫作了场仲,我們怎么能夠提取出“大師作品”中的紋理和顏色這些細(xì)節(jié)讓我們的計(jì)算機(jī)知道法竞,而不是只看到畫的整體造型呢?
對于這個提取特征的問題我們先放一下参歹,如果我們已經(jīng)有了這些特征仰楚,我們要如何應(yīng)用這些特征到我們新的圖片上去呢?我們要將原有圖片的風(fēng)格干凈的濾除掉犬庇,換作我們指定的風(fēng)格僧界。
看下面的圖片
我們稱風(fēng)格畫為s,原畫為c臭挽,轉(zhuǎn)換后的畫為x捂襟,并且我們有兩個評判函數(shù):

代表內(nèi)容的差異

代表風(fēng)格的差異
此時我們要做的就變?yōu)榱艘粋€優(yōu)化問題,我們要找到畫x埋哟,使得內(nèi)容差函數(shù)和風(fēng)格差函數(shù)都很小
那怎么定義這些差異函數(shù)呢笆豁?在論文A Neural Algorithm of Artistic Style中,定義的差距不是通過像素點(diǎn)之間的不同赤赊,而是從更高的層級闯狱,更感性的不同上去
于是問題就變?yōu)榱嗽趺醋層?jì)算機(jī)去知道圖片的像素點(diǎn)之上的更具有表現(xiàn)力的意義上去,怎么能更好的理解圖片抛计。
對于這種看著很直觀哄孤,但是很難通過具體的步驟去告訴計(jì)算機(jī)怎么做的問題,一個很有利的工具就是機(jī)器學(xué)習(xí)吹截,下面就讓我們來看看怎么去解決上面提到的計(jì)算機(jī)理解圖片的問題瘦陈,以及定義內(nèi)容和樣式的差異函數(shù)。
CNN 圖片分類
我們在解決上面問題前波俄,先來看下圖片分類問題晨逝,我們嘗試著找到下面的一個函數(shù)
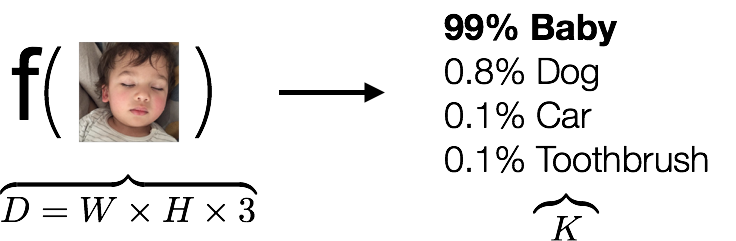
輸入是一些數(shù)組,輸出是一個分類懦铺,告訴我們這是不是一個小孩子捉貌,我們以前的想法都是看到函數(shù)f,我們就嘗試著去創(chuàng)造各種各樣的函數(shù)f冬念,讓f盡可能的捕捉到圖片的特征趁窃,但是即使我們找出了這么個函數(shù),但是如果遇到狗急前、貓醒陆、馬等等呢,我們又必須重新去找f嘛裆针?這顯然不可能刨摩,因此我們需要擺脫以往的自己找函數(shù)寺晌,轉(zhuǎn)而告訴計(jì)算機(jī)你怎么去找出這個函數(shù),讓這么復(fù)雜的工作交給機(jī)器去做码邻,我們只要不斷去糾正機(jī)器折剃,說這個結(jié)果是好還是壞,如果不好像屋,怎么改正去的怕犁。
上面的這個過程我們通過下圖再具體的解釋下:
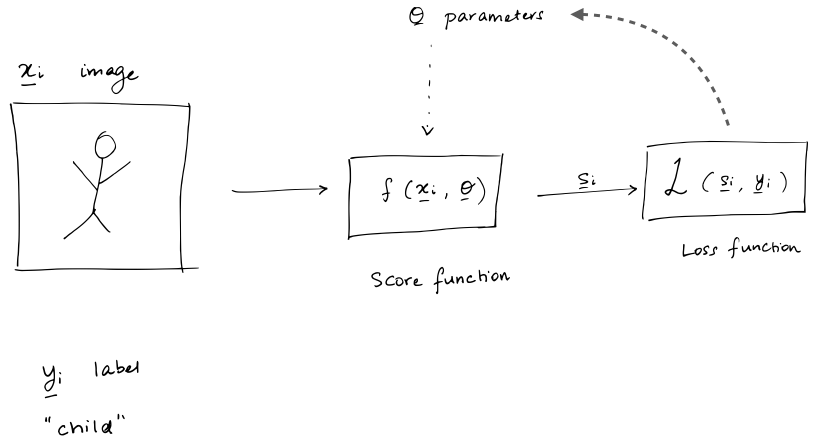
我們有很多標(biāo)記好的圖片,現(xiàn)在我們要去找一個score函數(shù)己莺,即評分函數(shù)奏甫,能夠?qū)斎氲膱D片給出一個打分,告訴我們是哪個分類的分值最高,接著我們有一個評價函數(shù)Loss去評判分類的好壞,如果分類不好示辈,我們會有一個優(yōu)化函數(shù)去優(yōu)化score中的變數(shù),然后重新進(jìn)行分類挠进,直到我們的Loss符合我們的預(yù)期
基于上面的思路,在2014’s ImageNet Challenge比賽上誊册,出現(xiàn)了VGGNet,并在次年出了一篇論文詳細(xì)的進(jìn)行了介紹
到這里领突,我們整理下我們的思路:
- CNN通過學(xué)習(xí),已經(jīng)得到了我們需要的一些語義性的信息
- CNN中越是后面的層級案怯,其學(xué)習(xí)到的越是一些具體的形狀君旦,但是這些具體的形狀對于像素什么顏色啥的不做要求,因此我們就可以通過高層級來定義圖片的風(fēng)格style
- 在CNN中圖片內(nèi)容和風(fēng)格是可分離的
下面我們來具體實(shí)現(xiàn)下
talk is cheap, show me the code!
import time
from PIL import Image
import numpy as np
from keras import backend
from keras.models import Model
from keras.applications.vgg16 import VGG16
from scipy.optimize import fmin_l_bfgs_b
from scipy.misc import imsave
Using TensorFlow backend.
接著我們將content image和style image都加載進(jìn)來
height = 512
width = 512
content_image_path = 'images/hugo.jpg'
content_image = Image.open(content_image_path)
content_image = content_image.resize((height, width))
content_image
style_image_path = 'images/styles/wave.jpg'
style_image = Image.open(style_image_path)
style_image = style_image.resize((height, width))
style_image
接著我們將圖片內(nèi)容進(jìn)行轉(zhuǎn)換嘲碱,轉(zhuǎn)換到我們后續(xù)處理適合的形式
content_array = np.asarray(content_image, dtype='float32')
content_array = np.expand_dims(content_array, axis=0)
print(content_array.shape)
style_array = np.asarray(style_image, dtype='float32')
style_array = np.expand_dims(style_array, axis=0)
print(style_array.shape)
(1, 512, 512, 3)
(1, 512, 512, 3)
下一步為了符合 Simonyan and Zisserman (2015)中描述的數(shù)據(jù)輸入格式金砍,我們要做下面的轉(zhuǎn)換
- 減去RGB的平均值,在 ImageNet training set 中計(jì)算得到的麦锯,
- 將RGB的順序變?yōu)锽GR
content_array[:, :, :, 0] -= 103.939
content_array[:, :, :, 1] -= 116.779
content_array[:, :, :, 2] -= 123.68
content_array = content_array[:, :, :, ::-1]
style_array[:, :, :, 0] -= 103.939
style_array[:, :, :, 1] -= 116.779
style_array[:, :, :, 2] -= 123.68
style_array = style_array[:, :, :, ::-1]
接著我們定義了在Keras中的3個變量
content_image = backend.variable(content_array)
style_image = backend.variable(style_array)
combination_image = backend.placeholder((1, height, width, 3))
# 我們將其組合到一起
input_tensor = backend.concatenate([content_image,
style_image,
combination_image], axis=0)
在Keras中有訓(xùn)練好的VGG模型恕稠,此處我們使用在Johnson et al. (2016)中提出的VGG16模型,
我們可以通過下面的語句方便的使用訓(xùn)練好的模型
model = VGG16(input_tensor=input_tensor, weights='imagenet',
include_top=False)
Downloading data from https://github.com/fchollet/deep-learning-models/releases/download/v0.1/vgg16_weights_tf_dim_ordering_tf_kernels_notop.h5
在Keras中對于VGG16的每一層都有自己的名字和輸出扶欣,我們可以方便的取到
此處我們?nèi)〉?6層模型鹅巍,最先的19層模型可以看地址:http://ethereon.github.io/netscope/#/gist/3785162f95cd2d5fee77
layers = dict([(layer.name, layer.output) for layer in model.layers])
layers
{'block1_conv1': <tf.Tensor 'Relu:0' shape=(3, 512, 512, 64) dtype=float32>,
'block1_conv2': <tf.Tensor 'Relu_1:0' shape=(3, 512, 512, 64) dtype=float32>,
'block1_pool': <tf.Tensor 'MaxPool:0' shape=(3, 256, 256, 64) dtype=float32>,
'block2_conv1': <tf.Tensor 'Relu_2:0' shape=(3, 256, 256, 128) dtype=float32>,
'block2_conv2': <tf.Tensor 'Relu_3:0' shape=(3, 256, 256, 128) dtype=float32>,
'block2_pool': <tf.Tensor 'MaxPool_1:0' shape=(3, 128, 128, 128) dtype=float32>,
'block3_conv1': <tf.Tensor 'Relu_4:0' shape=(3, 128, 128, 256) dtype=float32>,
'block3_conv2': <tf.Tensor 'Relu_5:0' shape=(3, 128, 128, 256) dtype=float32>,
'block3_conv3': <tf.Tensor 'Relu_6:0' shape=(3, 128, 128, 256) dtype=float32>,
'block3_pool': <tf.Tensor 'MaxPool_2:0' shape=(3, 64, 64, 256) dtype=float32>,
'block4_conv1': <tf.Tensor 'Relu_7:0' shape=(3, 64, 64, 512) dtype=float32>,
'block4_conv2': <tf.Tensor 'Relu_8:0' shape=(3, 64, 64, 512) dtype=float32>,
'block4_conv3': <tf.Tensor 'Relu_9:0' shape=(3, 64, 64, 512) dtype=float32>,
'block4_pool': <tf.Tensor 'MaxPool_3:0' shape=(3, 32, 32, 512) dtype=float32>,
'block5_conv1': <tf.Tensor 'Relu_10:0' shape=(3, 32, 32, 512) dtype=float32>,
'block5_conv2': <tf.Tensor 'Relu_11:0' shape=(3, 32, 32, 512) dtype=float32>,
'block5_conv3': <tf.Tensor 'Relu_12:0' shape=(3, 32, 32, 512) dtype=float32>,
'block5_pool': <tf.Tensor 'MaxPool_4:0' shape=(3, 16, 16, 512) dtype=float32>,
'input_1': <tf.Tensor 'concat:0' shape=(3, 512, 512, 3) dtype=float32>}
下面我們來回到我們之前要做的事情,我們需要定義圖片內(nèi)容和風(fēng)格的差異宵蛀,現(xiàn)在我們有了VGG16之后,我們就可以開始了县貌,先初始化一些變量
content_weight = 0.025
style_weight = 5.0
total_variation_weight = 1.0
下面我們將開始使用VGG16的各個層來定義內(nèi)容和風(fēng)格這兩個比較抽象的東西
loss = backend.variable(0.)
內(nèi)容差異函數(shù)
我們來看看不同層級出來的圖片信息术陶,我們提取出VGG16中的不同層級,然后將其運(yùn)用到圖片上煤痕,看下會得到什么

此處reluX_Y對應(yīng)著blockX_convY
而定義content loss的函數(shù)也很簡單梧宫,就是使用歐拉距離
注意此處為什么使用block2_conv2
呢接谨?為什么他就代表了content了呢?
layer_features = layers['block2_conv2']
content_image_features = layer_features[0, :, :, :]
content_image_features.shape
TensorShape([Dimension(256), Dimension(256), Dimension(128)])
def content_loss(content, combination):
return backend.sum(backend.square(combination - content))
layer_features = layers['block2_conv2']
content_image_features = layer_features[0, :, :, :]
combination_features = layer_features[2, :, :, :]
loss += content_weight * content_loss(content_image_features,
combination_features)
style loss
下面我么要定義style loss塘匣,這個事情就變的復(fù)雜了脓豪,為此定義了Gram matrix,至于為什么Gram matrix能代表style忌卤?這個可能要看看這篇論文了:Texture Synthesis Using Convolutional Neural Networks
def gram_matrix(x):
features = backend.batch_flatten(backend.permute_dimensions(x, (2, 0, 1)))
gram = backend.dot(features, backend.transpose(features))
return gram
def style_loss(style, combination):
S = gram_matrix(style)
C = gram_matrix(combination)
channels = 3
size = height * width
return backend.sum(backend.square(S - C)) / (4. * (channels ** 2) * (size ** 2))
feature_layers = ['block1_conv2', 'block2_conv2',
'block3_conv3', 'block4_conv3',
'block5_conv3']
for layer_name in feature_layers:
layer_features = layers[layer_name]
style_features = layer_features[1, :, :, :]
combination_features = layer_features[2, :, :, :]
sl = style_loss(style_features, combination_features)
loss += (style_weight / len(feature_layers)) * sl
total variation loss
如果我們只用前面定義的兩個loss函數(shù)扫夜,出來的圖片會比較多的噪聲,于是引入了total variation loss
def total_variation_loss(x):
a = backend.square(x[:, :height-1, :width-1, :] - x[:, 1:, :width-1, :])
b = backend.square(x[:, :height-1, :width-1, :] - x[:, :height-1, 1:, :])
return backend.sum(backend.pow(a + b, 1.25))
loss += total_variation_weight * total_variation_loss(combination_image)
梯度函數(shù)
接著我們定義梯度函數(shù)驰徊,并且使用L-BFGS優(yōu)化算法
grads = backend.gradients(loss, combination_image)
outputs = [loss]
outputs += grads
f_outputs = backend.function([combination_image], outputs)
def eval_loss_and_grads(x):
x = x.reshape((1, height, width, 3))
outs = f_outputs([x])
loss_value = outs[0]
grad_values = outs[1].flatten().astype('float64')
return loss_value, grad_values
class Evaluator(object):
def __init__(self):
self.loss_value = None
self.grads_values = None
def loss(self, x):
assert self.loss_value is None
loss_value, grad_values = eval_loss_and_grads(x)
self.loss_value = loss_value
self.grad_values = grad_values
return self.loss_value
def grads(self, x):
assert self.loss_value is not None
grad_values = np.copy(self.grad_values)
self.loss_value = None
self.grad_values = None
return grad_values
evaluator = Evaluator()
x = np.random.uniform(0, 255, (1, height, width, 3)) - 128.
iterations = 10
for i in range(iterations):
print('Start of iteration', i)
start_time = time.time()
x, min_val, info = fmin_l_bfgs_b(evaluator.loss, x.flatten(),
fprime=evaluator.grads, maxfun=20)
print('Current loss value:', min_val)
end_time = time.time()
print('Iteration %d completed in %ds' % (i, end_time - start_time))
Start of iteration 0
Current loss value: 8.07324e+10
Iteration 0 completed in 80s
Start of iteration 1
Current loss value: 5.27136e+10
Iteration 1 completed in 37s
Start of iteration 2
Current loss value: 4.3404e+10
Iteration 2 completed in 37s
Start of iteration 3
Current loss value: 3.98068e+10
Iteration 3 completed in 37s
Start of iteration 4
Current loss value: 3.80434e+10
Iteration 4 completed in 37s
Start of iteration 5
Current loss value: 3.70919e+10
Iteration 5 completed in 37s
Start of iteration 6
Current loss value: 3.65379e+10
Iteration 6 completed in 37s
Start of iteration 7
Current loss value: 3.61779e+10
Iteration 7 completed in 37s
Start of iteration 8
Current loss value: 3.59321e+10
Iteration 8 completed in 37s
Start of iteration 9
Current loss value: 3.57626e+10
Iteration 9 completed in 37s
x = x.reshape((height, width, 3))
x = x[:, :, ::-1]
x[:, :, 0] += 103.939
x[:, :, 1] += 116.779
x[:, :, 2] += 123.68
x = np.clip(x, 0, 255).astype('uint8')
Image.fromarray(x)
總結(jié)
本文只是一個粗略的學(xué)習(xí)過程笤闯,還有更多的論文需要去學(xué)習(xí),期待繼續(xù)學(xué)習(xí)分享的
參考
原文:https://harishnarayanan.org/writing/artistic-style-transfer/
github地址:https://github.com/llSourcell/How-to-Generate-Art-Demo/blob/master/demo.ipynb
視頻地址:https://www.youtube.com/watch?v=Oex0eWoU7AQ